This is a guest post from Luigi Muzii which is basically made up primarily of the speaker notes of his presentation at the ELIA Together 2018 conference. I believe that Luigi has wise words and represent best practices thinking and thus offer it on this blog forum.
While some of his advice might seem obvious to some, I am always struck by often these very commonsensical recommendations are either overlooked or simply ignored willfully.
As generic MT improves, I think it becomes more and more important for enterprises to consider bringing rampant, uncontrolled MT use by employees and outsourced translators under control.
As always the emphasis of bold text in the post is my doing.
====
The presentation was designed to provide some practical advice about tackling the challenges that freelancers, project managers, and translation buyers face when approaching MT, implementing MT or running MT and post-editing projects.
Three target groups can be identified for three kinds of task:
- In the making; machine translation is used for “suggestions” while processing a document by a human translator; this is probably the most common approach today;
- Downstream; machine translation is used to fully process a document that will be possibly post-edited; this approach is typically adopted by larger clients with established experience in the field;
- On constraints; machine translation is used by an LSP to finalize a translation job by asking translators to work on suggestions coming from a specialized engine.
Laying foundations
The three scenarios just outlined require different strategies to be devised. The first one involves the machine-translation method.Given the circumstances, the premises and the many reservations about it—basically, the hype—a question must be asked: Is PEMT already in the past?
In this paper, the reference method is PB-SMT (Phrase-Based Statistical Machine Translation) because PB-SMT engines are inexpensive and effective, whereas customizable NMT (Neural Machine Translation) engines are still quite pricey and challenging as to technical requirements and operational complexity, thus out of range for most customers. Translators working on unrestricted documents (scenario one applies here,) would generally choose an online NMT engine. For customers requiring confidentiality and IP protection and willing to leverage their own language data (scenario two and three apply here,) a highly customizable PB-SMT engine might be a valid option, especially where no major investment is envisaged in the field, vast and suitable data is available and/or limited volumes are processed.
In general, the main drivers in the adoption of MT are productivity (speed and volumes) and usefulness (consistency and marginal cost) especially for large projects otherwise involving many translators. Unfortunately, MT is not exactly child’s play. MT engines are complex and challenging applications requiring a combination of tools, data, and knowledge. This is a rare commodity, especially in a single person, on both sides of a translation project.
Also, in the future, while MT will continue to proliferate, the shortage of good translators will increase.
Join forces
Therefore, joining forces is important to explore and vet as many solutions as possible. According to a popular saying, everyone’s needed, no one’s indispensable, and can be easily replaced by anyone else with a similar profile in the same role. This also means, though, that, to be valuable, everyone’s effort is needed, on the highest level of performance all the time. For quite some time now, translation is no longer a solitary feat, but a collaborative effort. This is especially true with the current level of technology.In this respect, three steps should be completed before starting any MT project.
- Recap your goals and requirements
What you expect from MT and how much you are willing to rely on it. - Check your MT readiness;
Realistically analyze your knowledge, tools, and data. - Plan for assistance
Never venture into an unfamiliar territory without a guide.
Defining requirements
When planning to implement MT, keep scope, goals, and expectations clearly distinct.Identify one or more items within your scope of business for MT, possibly picking those where the amount of data available is larger, and the quality higher.
Clearly define and prioritize your goals. Major goals may be reducing labor, boosting productivity and keeping consistency, especially in larger projects.
Be realistic in your expectations. Therefore, familiarize with technology and strengthen your expertise to make the best of it. Tackle any security issues for confidentiality and data integrity and protection of intellectual property. Don’t forget to scrub your data if you plan to train an engine and to plan for any relevant support. Finally, revise your pricing model to encompass MT-related tasks.
Building a platform
When building an MT platform, never forget that MT engines are not all equal, for different environments, configurations, and data. Therefore, although the output could be considered someway predictable, raw output quality can vary across systems and language combinations, and error may not follow a consistent pattern. Performances of MT engines also vary.In data-driven MT, data maintenance is crucial, and it is the first task when setting up an MT platform. Data must be organized, cleaned, and fixed for terminology and style. For a customized engine, at least 100,000 paired segments are necessary and the cleaner and healthier the better.
Another important factor to the effectiveness of an MT strategy are the tools used for data preparation, pre-translation, and post-editing. Special attention must be given to translation tool settings to allow for sub-segment recall and fuzzy match repair.
Finally, when choosing the engine, the items to consider are:
- The total cost of ownership (TCO)
- Integration
- Expertise
- Security (especially as to intellectual property and confidentiality)
Best practices: Running projects
When running MT projects, best practices may be different depending on whether you are a translation buyer or vendor.In general, knowing your data and mastering quality metrics is a must. As to post-editing, devise your own compensation scheme.
A common mistake is to consider all content as equal and then mess with data. In the same way, absolutely avoid relying only on your capacities or on vendors. In the end, everything can be summed up in the simple invitation to not expect any miracles.
Never forget to agree with the customer and the content owner about using MT, especially if you are using a SaaS/online platform to prevent being sued.
Also, remember that data is the fuel to any SMT/NMT engine and that the output is only as good as the data used. In fact, these engines perform statistical predictions by inference, and when the amount and quality of data increase, an engine improves.
Collect as much data as possible, but always make sure it comes from few reliable sources in a restricted domain, that it contains correct translations with no errors, it is real and recent, and terminologically and stylistically consistent.
At this point, you must accept that MT output is unpredictable. For this reason, MT quality assessment should be run in such a way as to prevent any subjectivity.
For the same reason, post-editing is and will remain a critical, integrated part of MT usage, and it is expected to be fast, unchallenging, and flowing.
Anyway, the amount of post-editing required can be hard to assess. To plan deadlines and allocate a budget for the job, two different measures can be used, the edit time and the post-editing effort. The first is the time required to get a raw MT output to the desired standard, and the latter is the percentage of edits to be applied to raw MT output to attain the desired standard.
The main problem with edit time is that it can only be computed downstream, assuming that the time spent has been entirely devoted to editing.
The post-editing effort can be estimated through probabilistic forecasts based on automatic metrics as a reverse projection of the productivity boost. In fact, translation productivity is measured as the throughput expressed in the number of words per hour, and MT is supposed to improve it by reducing the turnaround time and increasing the workable volumes. However, the post-editing effort and the turnaround time are hard to predict, especially for translation of publishable quality and/or data for incremental training of engines. In fact, it depends on diverse factors such as the quality expectations for the finalized output, the volume of content to process, and the allotted time for the task. Also, the effort required depends on the post-editing level.
The post-editing level is generally restricted to:
- Gisting;
- Light;
- Full.
Finally, always follow a few basic rules before boarding on a post-editing project:
- Test before operating;
- Ask for MT samples for negotiation;
- Negotiate throughput rates;
- Ask for glossary with the list of DNT words;
- Ask for instructions;
- Be open to feedback.
- Never use MT to sustain price competition;
- Never process poor MT outputs;
- Never treat post-editing as fuzzy matches.
As to pricing and compensation, for light post-editing of very good output when speed is a major concern and the first requirement, a model should be settled prior to any assessment of the actual MT output based on a clear-cut predictive scheme. However, do not follow any translation-memory fuzzy matches scheme. In fact, while fuzzy matches over 85% are inherently correct and generally require minor changes, machine-translated segments may contain errors and inaccuracies, and even a supposedly light post-editing may prove challenging. A downstream computation scheme might also be devised in full post-editing for an accurate measurement of the actual work performed. This is usually made by computing the edit distance and then inferring the percentage on the hourly rate.
A negotiation grid can be helpful to cross-reference type and nature of engines, quality of raw output, and all the relevant technical requirements with compensation based on productivity, type of performance, bid (hourly rate) and ancillary services (e.g. filling in QA forms for ongoing training of engine.)
In any case, a strong and clear “No, thanks!” is more than reasonable when a considerably low pay rate is offered that is unrelated to language pair and MT output quality and/or MT output quality is lower than a generic free online service.
Lastly, raw MT output should be processed before a post-editing job for automatic removal of empty and/or untranslated segments and duplications, fixing of diacritics, punctuation marks, extra spaces, noise, and numbers; terminology should also be checked for consistency and a spellcheck should be run. A post-processing stage should also be envisaged involving encoding, normalization, formatting (especially tag injection,) a terminology check and, obviously, a spellcheck.
=======================
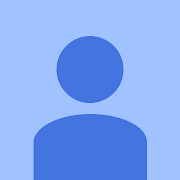
Luigi Muzii has been in the "translation business" since 1982 and has been a business consultant since 2002, in the translation and localization industry through his firm. He focuses on helping customers choose and implement best-suited technologies and redesign their business processes for the greatest effectiveness of translation and localization related work.
This link provides access to his other blog posts.
I like your scenario 1 (occasional use by human translators), but I don't see any real consideration of the advantages and drawbacks of this approach. Perhaps this indicates that it is actually a completely different industry from the mass market processing of multilingual content by major international corporations, and therefore not really within the remit of this blog.
ReplyDeleteI also appreciate your observation that many so-called "LSPs" (a.k.a. agencies) use MT and PEMT purely for price reasons. I would suggest that this is a major reason why many freelance translators will never trust PEMT.