This is a ranking of the most popular posts of 2016 on this blog according to Blogger, based on traffic and/or reader comment activity. Popular does not necessarily mean the best, and I have seen in the past that some posts that may not initially resonate, have real staying power and continue to be read actively years after the original publishing date, even though they are not initially popular. We can see from these rankings, that Neural MT certainly was an attention grabber for 2016, (even though I think for the business translation industry, Adaptive MT is a bigger game changer) and I look forward to seeing how NMT becomes more fitted to translation industry needs over the coming year.
I know with some certainty that the posts by Juan Rowda and Silvio Picinini will be read actively through the coming year and on, because they are not just current news that fades quickly like the Google NMT critique, but rather they are carefully gathered best practice knowledge that is useful as a reference over an extended period. These kinds of posts become long-term references that provide insight and guidance for others traversing a similar road or trying to build up task-specific expertise and wish to draw on best practices.
I have been much more active surveying the MT landscape since achieving my independent status, and I have a much better sense for the leading MT solutions now than I ever have.
This is a critique of the experimental process and related tremendous "success" reported by Google in making the somewhat outrageous claim that they had achieved "close to human translation" with their latest Neural MT technology. It is quite possible that the research team tried to rein in the hyperbole but were unsuccessful and the marketing team ruled on how this would be presented to the world.
This was an annual wrap-up of the year in MT. I was surprised by how actively this was shared and distributed and at the time of this post is still the top post as per the Google ranking system. The information in the post was originally done together as a webinar with Tony O'Dowd of KantanMT. It was also interesting for me as I did some research on how much MT is being used and found out that on any given day as much as 500+ Billion words a day are being passed through a public MT engine somewhere in the world.
This is yet another guest post, this time jointly with Luigi Muzii, that rapidly rose and gained visibility, as it provided some deeper analysis, and hopefully a better understanding of why private equity firms have focused so hard on the professional translation industry. There is a superficial reaction by many in the industry that seems to interpret this investment interest by PE firms as being so bullish on "translation," that they are interested in funding expansion plans at lackluster LSP candidates. A deeper examination, suggests that the investment clearly is not just to give money to the firms they invest in, but it appears that many large LSPs are good "business turnaround and improve" candidates. This suggests that one of these "improved" PE LSP investments could become a real trailblazer in terms of re-defining the business translation value equation, and begin a evolutionary process whereby many marginal LSPs could be driven out of the market. However, we have yet to see even small signs of real success by any of the PE supervised firms thus far in changing and upgrading the market dynamics.
The single most frequently read post I have written thus far, is one that focuses on post-editing compensation. It was written in early 2012, but to this day, it still gets at least 1,000 views a month. This, I suppose shows that the industry has not solved basic problems, and I noticed that I am still talking about this issue in my outlook on MT in 2017. It remains an issue that many have said needs a better problem resolution. Let's hope that we can develop much more robust approaches to this problem this year. As I have stated before, there is an opportunity for industry collaboration to develop and share actual work related data to develop more trusted measurements. If multiple agencies collaborate and share MT and PEMT experience data we could get to a point where the measurements are much more meaningful and consistent across agencies.
I know with some certainty that the posts by Juan Rowda and Silvio Picinini will be read actively through the coming year and on, because they are not just current news that fades quickly like the Google NMT critique, but rather they are carefully gathered best practice knowledge that is useful as a reference over an extended period. These kinds of posts become long-term references that provide insight and guidance for others traversing a similar road or trying to build up task-specific expertise and wish to draw on best practices.
I have been much more active surveying the MT landscape since achieving my independent status, and I have a much better sense for the leading MT solutions now than I ever have.
So here is the ranking of the most popular/active posts over the last 12 months.
The Google Neural Machine Translation Marketing Deception
This is a critique of the experimental process and related tremendous "success" reported by Google in making the somewhat outrageous claim that they had achieved "close to human translation" with their latest Neural MT technology. It is quite possible that the research team tried to rein in the hyperbole but were unsuccessful and the marketing team ruled on how this would be presented to the world.
A Deep Dive into SYSTRAN’s Neural Machine Translation (NMT) Technology
This is a report of a detailed interview with the SYSTRAN NMT team on their emergent neural MT technology. This was the first commercial vendor NMT solution available this last year and the continued progress looks very promising.This was an annual wrap-up of the year in MT. I was surprised by how actively this was shared and distributed and at the time of this post is still the top post as per the Google ranking system. The information in the post was originally done together as a webinar with Tony O'Dowd of KantanMT. It was also interesting for me as I did some research on how much MT is being used and found out that on any given day as much as 500+ Billion words a day are being passed through a public MT engine somewhere in the world.
This is a guest post by Silvio Picinini, a Machine Translation Language Specialist at eBay. The MTLS role is one that I think we will see more of within leading-edge LSPs as it simply makes sense when you are trying to solve large-scale translation challenges. The problems this eBay team solves have a much bigger impact on creating and driving positive outcomes for large-scale machine translation projects. The MTLS focus and approach is equivalent to taking post-editing to a strategic level of importance i.e. understand the data and solve 100,000 potential problems before an actual post-editor ever sees the MT output.
5 Tools to Build Your Basic Machine Translation Toolkit
This is another post from the MT Language Specialist team at eBay, by Juan Martín Fernández Rowda. This is a post that I expect will become a long-term reference article and I expect that it will be actively read even a year from now as it describes high-value tools that a linguist should consider when involved with large or massive scale translation project where MT is the only viable option. His other contributions are also very useful references and worth close reading.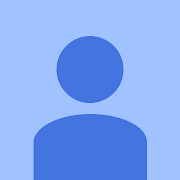
Comparing Neural MT, SMT and RBMT – The SYSTRAN Perspective
This is the result of an interesting interview with Jean Senellart, CEO and CTO at SYSTRAN, who is unique in the MT industry as being one of a handful of people who has deep exposure with all the current MT technology methodologies. In my conversations with Jean, I realized that he is one of the few people around in the "MT industry", who has deep knowledge and production-use experience with all three MT paradigms (RBMT, SMT, and NMT). There is a detailed article that describes the differences between these approaches on the SYSTRAN website for those who want more technical information.Jean Senellart, CEO, SYSTRAN SA
This was a guest post by Vladimir “Vova” Zakharov, the Head of Community at SmartCAT. It examines some of the most widely held misconceptions about computer-assisted translation (CAT) technology. SmartCAT is a very interesting new translation process management tool, that is free to use, and takes collaboration to a much higher level than I have seen with most other TMS systems. And interestingly, this post was also very popular in Russia.
The single most frequently read post I have written thus far, is one that focuses on post-editing compensation. It was written in early 2012, but to this day, it still gets at least 1,000 views a month. This, I suppose shows that the industry has not solved basic problems, and I noticed that I am still talking about this issue in my outlook on MT in 2017. It remains an issue that many have said needs a better problem resolution. Let's hope that we can develop much more robust approaches to this problem this year. As I have stated before, there is an opportunity for industry collaboration to develop and share actual work related data to develop more trusted measurements. If multiple agencies collaborate and share MT and PEMT experience data we could get to a point where the measurements are much more meaningful and consistent across agencies.
No comments:
Post a Comment