This is a guest post by Nico Herbig from the German Research Center for Artificial Intelligence (DFKI).
For as long as I have been involved with the translation industry, I have wondered why the prevailing translator machine interface was so arcane and primitive. It seems that the basic user interface used for managing translation memory was borrowed from DOS spreadsheets and has eventually evolved to become Windows spreadsheets. Apart from problems related to inaccurate matching, the basic interaction model has also been quite limited. Data enters the translation environment through some form of file or text import and is then processed in a columnar word processing style. I think to a great extent these limitations were due to the insistence on maintaining a desktop computing model for the translation task. While this does allow some power users to become productive keystroke experts it also presents a demanding learning curve to new translators.


Cloud-based translation environments can offer much more versatile and powerful interaction modes, and I saw evidence of this at the recent AMTA 2020 conference (a great conference by the way that deserves much better social media coverage than it has received.) Nico Herbig from the German Research Center for Artificial Intelligence (DFKI) presented a multi-modal translator environment that I felt shows great promise in updating the translator-machine interaction experience in the modern era.
Of course, it includes the ability to interact with the content via speech, handwriting, touch, eye-tracking, and seamless interaction with supportive tools like dictionaries, concordance databases, and MT among other possibilities. Nico's presentation focuses on the interface needs of the PEMT task, but the environment could be reconfigured for scenarios where MT is not involved and only used if it adds value to the translation task. I recommend that interested readers take a quick look through the video presentation to get a better sense of this.
*** ======== ***
MMPE: A Multi-Modal Interface for Post-Editing Machine Translation
As machine translation has been making substantial improvements in recent years, more and more professional translators are integrating this technology into their translation workflows. The process of using a
pre-translated text as a basis and improving it to create the final translation is called post-editing (PE). While PE can save time and reduce errors, it also affects the design of translation interfaces: the task changes from mainly generating text to correcting errors within otherwise helpful translation proposals, thereby requiring significantly less keyboard input, which in turn offers potential for interaction modalities other than mouse and keyboard. To explore which PE tasks might be well supported by which interaction modalities, we conducted a
so-called elicitation study, where participants can freely propose interactions without focusing on technical limitations. The results showed that professional translators envision PE interfaces relying on touch, pen, and speech input combined with mouse and keyboard as particularly useful. We thus developed and evaluated MMPE, a CAT
environment combining these input possibilities.
Hardware and Software
MMPE
was developed using web technologies and works within a browser. For handwriting support, one should ideally use a touch screen with a
digital pen, where larger displays and the option to tilt the screen or lay it on the desk facilitate ergonomic handwriting. Nevertheless, any tablet device also works. To improve automatic speech recognition accuracy, we recommend using an external microphone, e.g., a headset.
Mouse and keyboard are naturally supported as well. For exploring our newly developed eye-tracking features (see below), an eye tracker needs to be attached. Depending on the features to explore, a subset of this hardware is sufficient; there is no need to have the full setup. Since our focus is on exploring new interaction modalities, MMPE’s contribution lies on the front-end. At the same time, the backend is rather minimal,
supporting only storing and loading of files or forwarding the microphone stream to speech recognition services. Naturally, we plan on extending this functionality in the future, i.e., adding project and user management functionality, integrating Machine Translation (instead of loading it from file), Translation Memory, Quality Estimation, and other tools directly in the prototype.
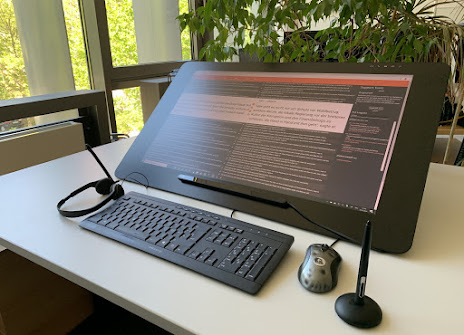
Interface Layout
As a layout, we implemented a horizontal source-target layout and tried to avoid overloading the interface. On the far right, support tools are offered,
e.g., a bilingual concordancer (Linguee). The top of the interface shows a
toolbar where users can save, load, and navigate between projects, and
enable or disable spell checking, whitespace visualization, speech
recognition and eye-tracking. The current segment is enlarged, thereby
offering space for handwritten input and allowing users to view the
context while still seeing the current segment in a comfortable manner.
The view for the current segment is further divided into the source
segment (left) and tabbed editing planes for the target (right), one for
handwriting and drawing gestures, and one for touch deletion &
reordering, as well as a standard mouse and keyboard input. By clicking on the tabs at the top, the user can quickly switch between the two modes. As the prototype focuses on PE, the target views initially show the MT proposal to be edited. Undo and redo functionality and segment confirmation are also implemented through hotkeys, buttons, or speech commands. Currently, we are adding further customization possibilities,
e.g., to adapt the font size or to switch between displaying source and target side by side or one above the other.
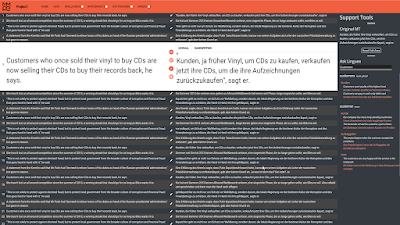
Handwriting
Hand-writing in the hand-writing tab is recognized using the MyScript Interactive Ink SDK,
which worked well in our study. The input field further offers drawing gestures like strike-through or scribble for deletions, breaking a word into two (draw a line from top to bottom), and joining words (draw a
line from bottom to top). If there is a lack of space to hand-write the intended text, the user can create such space by breaking the line (draw a long line from top to bottom). The editor further shows the recognized input immediately at the top of the drawing view. Apart from using the pen, the user can use his/her finger or the mouse for hand-writing, all of which have been used in our study, even though the pen was clearly preferred. Our participants highly valued deletion by strike-through or scribbling through the text, as this would nicely resemble standard copy-editing. However, hand-writing for replacements and insertions was considered to work well only for short modifications.
For more extended changes, participants argued that one should instead fall back to typing or speech commands.

Touch Reorder
Reordering using (pen
or finger) touch is supported with a simple drag and drop procedure:
Users have two options: (1) They can drag and drop single words by
starting a drag directly on top of a word, or (2) they can double-tap to
start a selection process, define which part of the sentence should be
selected (e.g., multiple words or a part of a word), and then move it.
We
visualize the picked-up word(s) below the touch position and show the calculated current drop position through a small arrow element. Spaces between words and punctuation marks are automatically fixed, i.e.,
double spaces at the pickup position are removed, and missing spaces at the drop position are inserted. In our study, touch reordering was highlighted as particularly useful or even “perfect” and received the highest subjective scores and lowest time required for reordering.

Speech
To minimize lag during
speech recognition, we use a streaming approach, sending the recorded
audio to IBM Watson servers to receive a transcription, which is then
interpreted in a command-based fashion. The transcription itself is
shown at the top of the default editing tab next to a microphone symbol.
As commands, post-editors can “insert,” “delete,” “replace,” and “reorder” words or sub-phrases. To specify the position if it is ambiguous, anchors can be specified, e.g., “after”/”before”/”between” or the occurrence of the token (“first”/”second”/”last”) can be defined. A full example is “replace A between B and C by D,” where A, B, C, and D can be words or sub-phrases. Again, spaces between words and punctuation marks are automatically fixed. In our study,
speech [recognition] received good ratings for insertions and replacements but worse ratings for reorderings and deletions. According to the participants, speech would become especially compelling for longer insertions and would be preferable when commands remain simple.
For invalid commands, we display why they are invalid below the transcription (e.g., “Cannot delete the comma after nevertheless, as nevertheless does not exist”).
Furthermore, the interface temporarily highlights insertions and replacements in green, deletions in red (the space at the position), and combinations of green and red for reorderings. The color fades away after the command.

Multi-Modal Combinations of Pen/Touch/Mouse&Keyboard with Speech
Multi-modal
combinations are also supported: Target word(s)/position(s) must first
be specified by performing a text selection using the pen, finger touch,
or the mouse/keyboard.
Afterwards, the user can use a voice command like “delete” (see the figure below), “insert A,” “move after/before A/between A and B,” or “replace with A”
without needing to specify the position/word, thereby making the
commands less complex. In our study, multi-modal interaction received
good ratings for insertions and replacements, but worse ratings for
reorderings and deletions.

Eye Tracking
While not tested in a
study yet, we currently explore other approaches to enhance PE through
multi-modal interaction, e.g., through the integration of an eye tracker.
The idea is to simply fixate the word to be replaced/deleted/reordered
or the gap used for insertion, and state the simplified speech command
(e.g., “replace with A”/”delete”), instead of having to
manually place the cursor through touch/pen/mouse/keyboard. To provide
feedback to the user, we show his/her fixations in the interface and
highlight text changes, as discussed above. Apart from possibly speeding
up multi-modal interaction, this approach would also solve the issue
reported by several participants in our study that one would have to “do
two things at once” while keeping the advantage of having simple
commands in comparison to the speech-only approach.

Logging
MMPE supports extensive logging functionality, where we log all text manipulations on a higher level to simplify text editing analysis. Specifically, we log whether the manipulation was an insertion, deletion, replacement, or reordering,
with the manipulated tokens, their positions, and the whole segment text. Furthermore, all log entries contain the modality of the interaction, e.g., speech or pen, thereby allowing the analysis of which modality was used for which editing operation.

Evaluation
Our study with professional translators showed a high level of interest and enthusiasm about using these new modalities. For deletions and reorderings, pen and touch both received high subjective ratings, with the pen being even better than the mouse & keyboard. Participants especially highlighted that pen and touch deletion or reordering “nicely resemble a
standard correction task.” For insertions and replacements, speech and multi-modal interaction of select & speech were seen as suitable interaction modes; however, mouse & keyboard were still favored and faster. Here, participants preferred the speech-only approach when commands are simple but stated that the multi-modal approach becomes relevant when the sentences' ambiguities make speech-only commands too complex. However, since the study participants stated that mouse and keyboard only work well due to years of experience and muscle memory,
we are optimistic that these new modalities can yield real benefit within future CAT tools.
Conclusion
Due to continuously
improving MT systems, PE is becoming more and more relevant in
modern-day translation. The interfaces used by translators still heavily
focus on translation from scratch, and in particular on mouse and
keyboard input modalities. Since PE requires less production of text but
instead requires more error corrections, we implemented and evaluated the MMPE
CAT environment that explores the use of speech commands, handwriting
input, touch reordering, and multi-modal combinations for PE of MT.
In the next steps, we want to run a study that specifically explores the
newly developed combination of eye and speech input for PE. Apart from
that, longer-term studies exploring how the modality usage changes over
time, whether translators continuously switch modalities or stick to
specific ones for specific tasks are planned.
Instead of
replacing the human translator with artificial intelligence (AI), MMPE
investigates approaches to better support the human-AI collaboration in
the translation domain by providing a multi-modal interface for
correcting machine-translation output. We are currently working on
proper code documentation and plan to open-source release the prototype
within the next months. MMPE was developed in a tight collaboration
between the German Research Center for Artificial Intelligence (DFKI) and Saarland University and is funded in part by the German Research Foundation (DFG).
Contact
Nico Herbig - nico.herbig@dfki.de
German Research Center for Artificial Intelligence (DFKI)
Further information:
Paper and additional information:
Multi-Modal Approaches for Post-Editing Machine Translation
Nico Herbig, Santanu Pal, Josef van Genabith, Antonio Krüger Proceedings of the 2019 CHI Conference on Human Factors in Computing Systems. ACM 2019
ACM Digital Library - Paper access
(Presenting an elicitation study that guided the design of MMPE)
MMPE: A Multi-Modal Interface using Handwriting, Touch Reordering, and Speech Commands for Post-Editing Machine Translation
Nico
Herbig, Santanu Pal, Tim Düwel, Kalliopi Meladaki, Mahsa Monshizadeh,
Vladislav Hnatovskiy, Antonio Krüger, Josef van Genabith Proceedings of the 58th Annual Meeting of the Association for Computational Linguistics: System Demonstrations. ACL 2020
ACL Anthology - Paper access
(Demo paper presenting the original prototype in detail)
MMPE: A Multi-Modal Interface for Post-Editing Machine Translation
Nico Herbig, Tim Düwel, Santanu Pal, Kalliopi Meladaki, Mahsa Monshizadeh, Antonio Krüger, Josef van Genabith Proceedings of the 58th Annual Meeting of the Association for Computational Linguistics. ACL 2020
ACL Anthology - Paper access - Video
(Briefly presenting MMPE prototype and focusing on its evaluation)
Improving the Multi-Modal Post-Editing (MMPE) CAT Environment based on Professional Translators’ Feedback
Nico Herbig, Santanu Pal, Tim Düwel, Raksha Shenoy, Antonio Krüger, Josef van Genabith Proceedings of the 1st Workshop on Post-Editing in Modern-Day Translation at AMTA 2020. ACL 2020
Paper access - Video of presentation
(Recent improvements and extensions to the prototype)