As one observes the continuing evolution of MT use in the professional translation industry, we see that we have reached a point where we have some useful insights about producing successful outcomes in our use of MT. From my perspective as a long-term observer and expert analyst of enterprise MT use, some of these include:
- Adaptation and customization of a generic MT engine done with expertise generally produces a better outcome than simply using a generic public MT system.
- Working with enhanced baseline engines built by experts is likely to produce better outcomes than dabbling with Open Source options with limited expertise. While it has gotten easier to produce MT systems with open-source platforms, real expertise requires long-term exposure and repeated experimentation.
- The algorithms underlying Neural MT have become largely commoditized and there is little advantage gained by jumping from one NMT platform to another.
- More data is ONLY better if it is clean, relevant, and applicable to the enterprise use case in focus. It can be said today that (training) data often matters more than the algorithms used, but data quality and organization is a critical factor for creating successful outcomes.
- A large majority of translators still view MT with great skepticism and see it as marginally useful, mostly because of repeated exposure to incompetently deployed MT systems that are used to reduce translator compensation. Getting active and enthusiastic translator buy-in continues to be a challenge for most MT developers and getting this approval is a clear indicator of superior expertise.
- Attempts to compare different MT systems are largely unsuccessful or misleading, as they are typically based on irrelevant test data or draw conclusions based on very small samples.
- A large number of enterprise use cases are limited by scarce training data resources and thus adaptation and customization attempts have limited success.
I have been skeptical of the validity of many of the comparisons we see of MT systems produced by LSPs and "independent" evaluators nowadays, because of the questionable evaluation methodologies used. The evaluators often produce nice graphics but just as often produce misleading results that need further investigation. However, these comparative evaluations of different MT systems can still be useful to get a rough idea of the performance of generic systems of these MT vendors. Over the last few years ModernMT has been consistently showing up amongst the top-performing MT systems in many different evaluations, and thus I decided to sit down with the ModernMT team to better understand their technology and product philosophy and understand what might be driving this consistent performance advantage. The level of transparency and forthcoming nature of the responses from the ModernMT team was refreshing in contrast to other conversations I have had with other MT developers.

The MT journey here began over 10 years ago with Moses and Statistical MT,
but unlike most other long-term MT initiatives I know of, this effort was very translator-centric right from its inception. The system was used heavily by translators who worked for
Translated and the MT systems were continually adapted and modified to meet the needs of production translators. This is a central design intention and it is important to not gloss over this, as this is the ONLY MT initiative I know of where Translator Acceptance is used as the primary criterion on an ongoing basis, in determining whether MT should be used for production work or not. The operations managers will simply not use MT if it does not add value to the production process and causes translator discontent. Over many years the ongoing collaboration with translators at ModernMT has triggered MT system and process development changes to reach the current status quo, where the MT value-add and efficiency is clear to all the stakeholders.
The long-term collaboration between translators and MT developers, and resulting system and process modifications are a key reason why ModernMT does so well in both generic MT system comparisons, and especially in adapted/customized MT comparisons.
Thus, translators who actively use the ModernMT platform do so most often through
MateCat, an open-source CAT tool that ties together
MyMemory
(a large free-access shared TM repository with around 50 billion words in it) together with ModernMT or other MT platforms. MT is presented to translators as an alternative to TM on a routine basis, and corrections are dynamically and systematically used to drive continuous improvements in the ModernMT engines. Trados and other CAT tools are also able to seamlessly connect to the ModernMT back-end but these systems may see less immediate improvements in the MT output quality. However, this has not stopped ~25,000 downloads of the ModernMT plugin for Trados on the SDL Appstore. Translators who do production work for Translated are often given a choice of using Google instead of ModernMT but most have learned that ModernMT output improves rapidly from corrective feedback and that collaborative input is also easier, and thus tend to prefer it as shown in the surveys below.
Over the years the ModernMT product evolution has been driven by changes to identify and reduce post-editing effort rather than optimizing BLEU scores as most others have done.
In contrast to most MTPE experiences, the individual translator experience here is characterized by the following:
- A close and symbiotic relationship between a relevant translation memory and MT, even at the translator UX level
- An MT system that is constantly updated and can potentially improve with every single interaction and unit of corrective feedback
- Immediate project startup possibilities as no batch MT training process is necessary
- Translator control over all steering data used in a project means very straightforward control over terminology and term consistency, mirroring the latest TMs and linguistic preferences
- Corrective feedback given to the MT system is dynamic and continuous and can have an immediate impact on the next sentence produced by the MT system
- One of very few MT systems available today that can provide a context-sensitive translation
- Measurable and palpable reduction in post-editing effort and translator UX compared to other MT platforms
- Continuing free access to the CAT tool needed to integrate MT with TM, and interact proactively with MT with the option to use other highly regarded CAT tools if needed.

Memory here refers to user input data TM and glossaries to tune the generic system to the needs of the current translation task
Instance-Based Adaptation
ModernMT describes itself as an "Instance-Based Adaptive MT" platform. This means that it can start adapting and tuning the MT output to the customer subject domain immediately, without a batch customization phase. There is no long-running (hours/days/weeks) data preparation and pre-training process needed upfront. There is also no need to wait and gather a sufficient volume of corrective feedback to update and improve the MT engine on an ongoing basis. It is learning all the time.
Rapid adaptation to customer-unique language and terminology is perhaps the single most critical requirement for a global enterprise, and thus this is an optimal design for enterprises that works optimally with their specialized and unique content. This is also true for LSPs too, for that matter. ModernMT can adapt the MT system with as little as a single sentence, though the results are better if more data is provided. The team told me that 100K words (10-12,000 sentences) would generally produce consistently good results that are superior to any generic engine. The long-term impact of this close collaboration with translators who provide ongoing corrections, feedback on critical requirements to improve efficiency and process workflow, and careful acquisition of the right kind of data, results in the kind of relative performance rankings that ModernMT now regularly sees as a matter of course. One might even go so far as to say that they have built a sustainable competitive advantage.
I have always felt that a properly designed Man-Machine collaboration would very likely outperform an MT design approach that relies entirely on algorithms and/or data alone. We can see this is true from the comparative results of the large public MT portals who probably have 100X or more of the resources and budget that ModernMT does.
The understanding of the translation task and resulting directives that ongoing translator feedback brings to the table is an ingredient that most current MT systems lack. Gary Marcus and other AI experts have been vocal in pointing out that machine learning and data alone is not the best way forward and more human steering and symbolic knowledge is needed for better outcomes.
Special Features
ModernMT is a context-aware machine translation product that learns from user corrections. There has recently been growing interest in the MT research community to bring a greater degree of contextual awareness to MT systems and ModernMT has also been investigating implementing capabilities around doing this. The current production version has an implementation of this already, and this feature continues to evolve in speed, efficiency, and capability.

The ModernMT Context Analyzer analyzes an entire document text to be translated in milliseconds before producing a translation. This analysis seeks out and identifies the distinctive terminology and intrinsic style of the document. This information is then used to automatically select the most suitable private translation memories loaded by the user for that particular document. This results in the engine selecting the translation memory inventory that best reflects the right terminology and writing style. It is precisely this inventory that the MT engine
leverages to customize the output in real-time, for each and every sentence of the document.
As translators at Translated working with ModernMT regularly have the ability to compare the MT output with that of Google Translate, the developers monitor translator preferences on an ongoing basis. This ensures that translators are always working with the MT output that they find most useful and that developers understand when their own engines need to be improved or enhanced. The following charts are based on feedback from translators during production work and show a very definite preference for the rapidly improving ModernMT engine output. This preference is seen in internal translator assessments working in production mode rather than just a selective test set, and this has also been confirmed by independent third-party assessments with both automated scores and human evaluations. They all consistently show that ModernMT customizations regularly outperform most others in independent comparative evaluations. The forces driving this superior performance are the result of design philosophy and long-term man-machine collaboration that cannot be easily replicated by others.


Recent comparative assessments done by independent third parties also confirm this preference using different evaluation methods that include both human and automated metrics as shown below. It is not unreasonable to presume that this performance advantage will remain intact for at least the short term.
Data Privacy
In response to a question on data privacy, Davide Caroselli, VP of Product, ModernMT responded: "Any content sent to ModernMT, whether a “TMX” memory or an MTPE correction
from a professional translator, is saved in the user’s private data area. In
fact, only you will be able to access your resources and make ModernMT
adjust to them; in no way will another user be able to utilize that
same inventory for his/her system, nor will ModernMT itself be able to
use those contents, other than to exclusively offer your personalized
translation service.
In addition, ModernMT uses state-of-the-art encryption technologies to provide its cloud services. Our data centers, employee processes and
office operations are ISO 27001:2013 certified."
On-Premise Capabilities
While the bulk of the current ModernMT customer base works with the secure cloud deployment, the team at ModernMT has also defined a range of on-premise deployment capabilities for those enterprises that need the security, control, and assured data privacy needs that characterizes some National Security, Financial, Legal, and Healthcare/Pharma industry requirements.
The open-source foundations of much of the ModernMT infrastructure should make it particularly interesting to US Government Intelligence and Law-Enforcement agencies seeking large-scale multilingual data processing capabilities for eDiscovery and Social Media Surveillance applications.
Given that ModernMT is a continuous learning MT platform that learns with each correction, dynamically, there is a requirement for more GPU infrastructure than some other on-premise solutions in the market. However, there is a strong focus on computational efficiency to minimize the IT footprint needed to deploy it on-premise, and based on information provided to me, their capabilities are quite similar to competitive alternatives both in terms of hardware requirements and software pricing. Hardware costs are linked to throughput expectations with more hardware required for high throughput requirements. As with most machine learning-intensive capabilities, only enterprises with competent IT teams could undertake this as an internal deployment, and most LSPs and localization departments will see a lower total cost of ownership with the cloud deployment.
Enterprise Readiness
As ModernMT has evolved from the localization world it is already optimized for MT use cases where there is a significant need for a machine-first human optimized approach. More and more we see this model as being a preferred approach for the exploding volumes of localization content. The Localization Use Case is possibly the most challenging MT use case out there, as it requires very high-quality initial output that translators are willing to work with where it can be proven that the MT enhances productivity and efficiency. Localization use cases demand the highest quality MT output from the outset compared to eDiscovery, social media surveillance, eCommerce, customer service & support use cases which are all more tolerant of lower MT output quality on much larger volumes of data. Very few MT developers have had success with the high-quality and rapid responsiveness needs of the localization use case and many have tried and failed. This is why LSP adoption of MT is so low. ModernMT's success with the challenging localization use case, however, positions them very well for other MT use cases as their growing success with these other use cases proves.
The
ASTW case study illustrates the success of ModernMT in Intellectual Property (Patents) and Life Science focused translations, where the ease of customization for complex terminology and morphology, the ability to learn continuously and quickly from corrective feedback, and superior MTPE experience compared to other MT solutions has quickly made it a preferred solution.
"ModernMT is currently our favorite MT engine, especially in patent translations and in the Life Science sector,
because it proves reliable, efficient, qualitatively better than its
competitors, easily customizable and advantageous in terms of cost." Domenico Lombardini, CEO ASTW
We see that
eCommerce giants understand the positive impact of translating huge volumes of catalog and user-generated CX content has on driving international revenue growth with the examples of eBay, Amazon, and Alibaba. ModernMT is now the MT engine driving the multilingual expansion of Airbnb web content and is translating many billions of words a month for them. User-generated content influences future customers, and there is great value in translating this content to drive and grow international business. Interestingly ModernMT began this initiative with almost no translation memory and had to perform specialized heuristic analysis on Airbnb content to build the training material.
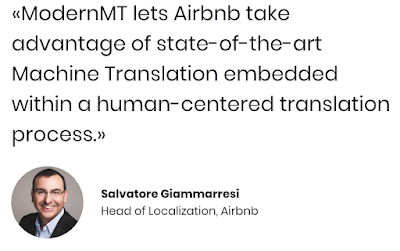
ModernMT has reached this point with very little investment in sales and marketing infrastructure. As this builds out and expands I will be surprised if ModernMT does not continue to expand and grow its enterprise presence, as enterprise buyers begin to understand that a tightly integrated man-machine collaborative platform that is continuously learning, is key to creating successful MT outcomes. I am aware that many other high-profile enterprise conversations are underway, and I expect that most enterprise buyers who evaluate the ModernMT platform will very likely find it is a preferred, cost-efficient way to implement large-scale MT solutions in a way that dramatically raises the likelihood of success.
Future Directions
Davide also mentioned to me that his team is very connected to the AI
community in Italy, and have been experimenting with GPT-3 and BERT, and
will continue to do so until clear value-added applications that
support and enhance their MT product emerge. ModernMT has a close
relationship with
Pi Campus and thus has regular interaction with luminaries in the AI community e.g. Lukasz Kaiser who will be speaking about
improvements in the Transformer architecture later this month.
The team also showed me demos of complex video content that had
ModernMT-based automated dubbing from English to Italian injected into
it. Apparently, Italy is one of the largest dubbing markets in the world. Who knew? Since my wife speaks Italian, I showed her some
National Geographic content on geology, filled with complex terminology and scientific subject matter that she was shocked to find out had been done completely without human modification. The Translated team is exploring Speech Translation and I expect that they will be quality leaders here too.
ModernMT
will continue to expand its connectivity to other translation and
content management infrastructure to make it easier to get
translation-worthy data in and out of their environment. They also
continue to explore ways to make the ModernMT continuous training
infrastructure more computationally efficient so that it can be more
easily deployed on smaller footprint hardware.
I
expect we will see more and more of ModernMT on the enterprise MT stage
from now on, as buyers realize that this is a significantly improved
next-generation MT solution that is more likely to produce successful
outcomes in digital transformation-related enterprise use scenarios. The
ModernMT approach reduces the uncertainty that is so common with most
MT-related initiatives and does it so seamlessly that most would not
realize how sophisticated the underlying technology is until they
attempt to replicate the functionality.
On a completely different note, I participated some months ago in responding to a question posed by Luca Di Biase, the
Imminent Research Director. He posed this same question to many luminaries in the translation industry, and also to me. The question has already triggered several
discussions on Twitter.
“Is language a technology or a culture?”
My response was as follows, but I think you may find the many other responses more interesting and complete if you go to this
link or look at some of
the other Twitter comments.
It is neither. Language is a means of communication and an information-sharing protocol that employs sounds, symbols, and gestures.
Language can sometimes use technology to enable amplification, extend the reach of messages, and accelerate information and knowledge sharing.
Language can create a culture when shared with(in) a group and used with well-understood protocols and norms. Intercultural communication
can also mean cross species, e.g., when communicating with dogs and horses.
Translated's Research Center has just
released the Imminent publication which has a distinctive style coupled with interesting content, that I think most in the language industry would find compelling and worth a close look.
Great article, thanks! Best part for me: "The resistance to MT is a perfect example of a missed opportunity. Instead of learning to use it better, in a more integrated, knowledgeable, and value-adding way for clients, it has become another badly used tool whose adoption struggles along, and MT use is most frequently associated with inflicting pain and low compensation on the translators forced to work with these sub-optimal systems".
ReplyDelete