This is a post by Luigi Muzii that was initially triggered by this post and this one, but I think it has grown into a broader comment on a key issue related to the successful professional use of MT i.e. the assessment of MT quality and the extent, scope, and management of the post-editing effort. Being able to get a quick and accurate assessment of the specific quality at any given time in a production use scenario is critical, but the assessment process itself cannot be so cumbersome and so complicated a process that the measurement effort becomes a new problem in itself.
While we see that industry leaders and academics continue to develop well meaning but very difficult to deploy (efficiently and cost-effectively) metrics like MQM and DQF, most practitioners are left with BLEU and TER as the only viable and cost-effective measures. However, these easy-to-do metrics have well-known bias issues with RbMT and now with NMT. And given that this estimation issue is the “crux of the biscuit” as Zappa would say, it is worth ongoing consideration and review as doing this correctly is where MT success is hidden.
Luigi's insistence on keeping this measurement simple, sometimes makes him unpopular with academics and industry "experts", but I believe that this issue is so often at the heart of a successful and unsuccessful MT deployment that it bears repeated exposure and frequent re-examination as we inch our way to more practical and useful measurement procedures than BLEU which continues to confound discussions of real progress in improving MT quality.
KISS - “Keep it simple, stupid” is a design principle noted by the U.S. Navy in 1960 stating that most systems work best if they are kept simple rather than made complicated.
The best way to understand the powers and limitations of a technology is to use it.
This can be easily shown for any general-purpose technology, and machine translation can now be considered as such. In fact, the major accomplishment we can acknowledge to Google Translate is that of having popularized widespread translation activity using machine translation, something most celebrated academics, and supposedly influential professional bodies have not been able to achieve after decades of trying.
The translation quality assessment debacle is emblematic i.e. the translation quality issue is, in many ways, representative of the whole translation community. It has been debated for centuries, mostly at conferences where insiders — always the same people — talk amongst themselves. And the people attending conferences of one kind do not talk with people attending conferences of another kind.
This ill-conceived approach to quality assessment has claimed victims even among scientists working on automatic evaluation methods. Just recently, the nonsensical notion of a “perfect translation” regained momentum. Everybody even fleetingly involved in translation should know that there is nothing easier to show as flawed than the notion of a “perfect translation”. At least according to current assessment practices, in a typical confirmation bias pattern. There is a difference between “acceptable” and “usable” translation and any notion of a perfect translation.
On the verge of the ultimate disruption, translation orthodoxy still dominates even the technology landscape by eradicating the key principle of innovation, simplicity.
The expected, and yet the overwhelming growth of content has long been going hand in hand with a demand for faster translation in an ever-growing number of language pairs, with machine translation being suggested as the one solution.
The issue remains unsolved, though, of providing buyers with an easy way to know whether the game is worth the candle. Instead, the translation community has been unable so far to provide unknowing buyers anything but an intricate maze of categories and error typologies, weights and parameters, where even an experienced linguist can have a hard time to find his way around.
The still largely widespread claim that the industry should “educate the client” is the concrete manifestation of the typical information asymmetry affecting the translation sector. By inadvertently keeping the customer in the dark, translation academics, pundits, and providers cuddle the silly illusion of gaining respect and consideration for their roles, while they are simply shooting themselves in the feet.
When KantanMT’s Poulomi Choudhury highlights the importance of the central role that the Multidimensional Quality Metrics (MQM) is supposed to play, in all likelihood she is talking to her fellow linguists. However, typical customers simply want to know whether they have to spend further to refine a translation and — possibly — understand how much. Typical customers who are ignorant of the translation production process are not interested in the kind of KPIs that Poulomi describes, while they could be interested in a totally different set of KPIs, to assess the reliability of a prospective partner.
Possibly, the perverse complexity of unnecessarily intricate metrics for translation quality assessment is meant to hide the uncertainty and resulting ambiguity of theorists and the inability and failure of theory rather than to reassure customers and provide them with usable tools.
In fact, every time you try to question the cumbersome and flawed mechanism behind such metrics, the academic community closes like a clam.
In her post, Poulomi Choudhury suggests setting exact parameters for reviewers. Unfortunately, the inception of the countless fights between translators and reviewers, between translators and reviewers and terminologists, and between translators and reviewers and terminologists and subject experts and in-country reviewers gets lost in the mist of time.
Not only are reviewing and post-editing (PEMT) instructions a rare commodity, the same translation pundits who tirelessly flood the industry with pointless standards and intricate metrics — possibly without having spent a single hour in their life negotiating with customers — have not produced even a guideline skeleton to help practitioners develop such procedural overviews.
As implementing a machine translation platform is no stroll for DIY ramblers, writing PEMT guidelines is not straightforward either, requiring specific know-how, and understanding, recalling the rationale for hiring a consultant when working with MT.
For example, although writing instructions for post-editors is a once-only task, different engines, domains, and language pairs require different instructions to meet the needs of different PEMT efforts. Once written, these instructions must then be kept up-to-date as new engines, language pairs, or domains are implemented so they vary continuously. Also, to help project managers assess the PEMT effort, these instructions should address the quality issue with guidelines and thresholds and scores for raw translation. Obviously, they should be clear and concise, and this might very well be the hardest part.
As well as being related to the quality of the raw output, the PEMT effort is a measure that any customer should be able to easily understand as a direct indicator of the potential expenditures to achieve business goals. In this respect, it should be properly described and we should go with tools that help the customer financially estimate the amount of work required to achieve the desired quality level from a machine translation output.
Indeed, the PEMT effort depends on diverse factors such as the volume of content to process, the turnaround time, and the quality expectations for the finalized output. Most importantly, it depends on the suitability of source data and input for (machine) translation.
Therefore, however, assessable through automatic measurements, PEMT effort can only be loosely estimated and projected. In this respect, KantanMT is offering the finest tool combination for accurate estimates.
On the other hand, a downstream measurement of the PEMT effort by comparing the final post-edited translation with the raw machine translation output is reactive (just like the typical translation quality assessment practice) rather than predictive (that is business-oriented).
Also, a downstream compensation model requires an accurate measurement of the actual work performed to infer the percentage on the hourly rate from the edit distance, as no positive correlation exists between edit distance and actual throughput.
Nonetheless, tracking the PEMT effort can be useful if the resulting data is compared with estimates to derive a historical series. After all, that’s how data empowers us.
Predictability is a major driver in any business, and it should come as no surprise, then, that translation buyers have no interest in dealing with the intricacy of quality metrics that are irredeemably prone to subjectivity, ambiguity, and misinterpretation, and, most importantly, are irrelevant to them. When it comes to business — and real money — gambling is never the first option, but the last resort. (KV: Predictability here would mean a defined effort ($ & time) that would result in a defined outcome (sample of acceptable output)).
On the other hand, more than a quarter of a century has passed since the introduction of CAT tools in the professional field, many books and papers have been written about them, and yet many still feel the urge to explain what they are. Maybe this might make sense for the few customers who are entirely new to translation, even though what they could be interested to know is just that providers would use some tool of the trade and spare them some money. And yet, quality would remain a major concern, as a recent SDL study showed.
An introduction to CAT tools is at the very least curious when recipients are translation professionals or translation students about to graduate. Even debunking some still popular myths about CAT is just as curious, unless considering the number of preachers thundering from their virtual pulpits against the hazards of these instruments of the devil.
In this apocalyptic scenario, even a significant leap forward could go almost unnoticed. Lilt is an innovative translation tool, with some fabulous features, especially for professional translators. As Kirti Vashee points out, it is a virtual translator assistant. It also presents a few drawbacks, though.
Post-editing is the ferry to the singularity. It could be run interactively, or downstream on an entire corpus of machine translation output.
When fed with properly arranged linguistic data from existing translation memories, Lilt could be an extraordinary post-editing tool also on bilingual files. Unfortunately, the edits made by a single user only affects the dataset associated with that account and the task that is underway. In other words, Lilt is by no means a collaborative translation environment. Yet.
This means that, for Lilt to be effective with typically large PEMT jobs involving teams, accurate PEMT instructions are essential, and, most importantly, post-editors should strictly follow them. This is a serious issue. Computers never break rules, while free-will enables humans to deviate from them.
Finally, although cloud computing is now usual in business, Lilt can still present a major problem to many translation industry players for being only available in the cloud, due to the need for a fast Internet connection, or to the vexed — although repeatedly demystified — question of data protection for IP reasons, and despite the computing resources to process the vast amount of data that would hardly make sense for a typical SME to have.
In conclusion, when you start a business, it is usually to make money, and money is not necessarily bad if you do no evil, pecunia non olet. And money usually comes from buyers, whose prime requirement can be summarized as “Give me something I can understand.”
Luigi Muzii has been in the "translation business" since 1982 and has been a business consultant since 2002, in the translation and localization industry through his firm . He focuses on helping customers choose and implement best-suited technologies and redesign their business processes for the greatest effectiveness of translation and localization related work.
This link provides access to his other blog posts.
While we see that industry leaders and academics continue to develop well meaning but very difficult to deploy (efficiently and cost-effectively) metrics like MQM and DQF, most practitioners are left with BLEU and TER as the only viable and cost-effective measures. However, these easy-to-do metrics have well-known bias issues with RbMT and now with NMT. And given that this estimation issue is the “crux of the biscuit” as Zappa would say, it is worth ongoing consideration and review as doing this correctly is where MT success is hidden.
Luigi's insistence on keeping this measurement simple, sometimes makes him unpopular with academics and industry "experts", but I believe that this issue is so often at the heart of a successful and unsuccessful MT deployment that it bears repeated exposure and frequent re-examination as we inch our way to more practical and useful measurement procedures than BLEU which continues to confound discussions of real progress in improving MT quality.
-------------------
The most profound technologies are those that disappear.
Mark Weiser
Mark Weiser
The Computer for the Twenty-First Century, Scientific American, 1991, pp. 66–75
The best way to understand the powers and limitations of a technology is to use it.
This can be easily shown for any general-purpose technology, and machine translation can now be considered as such. In fact, the major accomplishment we can acknowledge to Google Translate is that of having popularized widespread translation activity using machine translation, something most celebrated academics, and supposedly influential professional bodies have not been able to achieve after decades of trying.
The translation quality assessment debacle is emblematic i.e. the translation quality issue is, in many ways, representative of the whole translation community. It has been debated for centuries, mostly at conferences where insiders — always the same people — talk amongst themselves. And the people attending conferences of one kind do not talk with people attending conferences of another kind.
This ill-conceived approach to quality assessment has claimed victims even among scientists working on automatic evaluation methods. Just recently, the nonsensical notion of a “perfect translation” regained momentum. Everybody even fleetingly involved in translation should know that there is nothing easier to show as flawed than the notion of a “perfect translation”. At least according to current assessment practices, in a typical confirmation bias pattern. There is a difference between “acceptable” and “usable” translation and any notion of a perfect translation.
On the verge of the ultimate disruption, translation orthodoxy still dominates even the technology landscape by eradicating the key principle of innovation, simplicity.
The expected, and yet the overwhelming growth of content has long been going hand in hand with a demand for faster translation in an ever-growing number of language pairs, with machine translation being suggested as the one solution.
The issue remains unsolved, though, of providing buyers with an easy way to know whether the game is worth the candle. Instead, the translation community has been unable so far to provide unknowing buyers anything but an intricate maze of categories and error typologies, weights and parameters, where even an experienced linguist can have a hard time to find his way around.
The still largely widespread claim that the industry should “educate the client” is the concrete manifestation of the typical information asymmetry affecting the translation sector. By inadvertently keeping the customer in the dark, translation academics, pundits, and providers cuddle the silly illusion of gaining respect and consideration for their roles, while they are simply shooting themselves in the feet.
When KantanMT’s Poulomi Choudhury highlights the importance of the central role that the Multidimensional Quality Metrics (MQM) is supposed to play, in all likelihood she is talking to her fellow linguists. However, typical customers simply want to know whether they have to spend further to refine a translation and — possibly — understand how much. Typical customers who are ignorant of the translation production process are not interested in the kind of KPIs that Poulomi describes, while they could be interested in a totally different set of KPIs, to assess the reliability of a prospective partner.
Possibly, the perverse complexity of unnecessarily intricate metrics for translation quality assessment is meant to hide the uncertainty and resulting ambiguity of theorists and the inability and failure of theory rather than to reassure customers and provide them with usable tools.
In fact, every time you try to question the cumbersome and flawed mechanism behind such metrics, the academic community closes like a clam.
In her post, Poulomi Choudhury suggests setting exact parameters for reviewers. Unfortunately, the inception of the countless fights between translators and reviewers, between translators and reviewers and terminologists, and between translators and reviewers and terminologists and subject experts and in-country reviewers gets lost in the mist of time.
Not only are reviewing and post-editing (PEMT) instructions a rare commodity, the same translation pundits who tirelessly flood the industry with pointless standards and intricate metrics — possibly without having spent a single hour in their life negotiating with customers — have not produced even a guideline skeleton to help practitioners develop such procedural overviews.
As implementing a machine translation platform is no stroll for DIY ramblers, writing PEMT guidelines is not straightforward either, requiring specific know-how, and understanding, recalling the rationale for hiring a consultant when working with MT.
For example, although writing instructions for post-editors is a once-only task, different engines, domains, and language pairs require different instructions to meet the needs of different PEMT efforts. Once written, these instructions must then be kept up-to-date as new engines, language pairs, or domains are implemented so they vary continuously. Also, to help project managers assess the PEMT effort, these instructions should address the quality issue with guidelines and thresholds and scores for raw translation. Obviously, they should be clear and concise, and this might very well be the hardest part.
As well as being related to the quality of the raw output, the PEMT effort is a measure that any customer should be able to easily understand as a direct indicator of the potential expenditures to achieve business goals. In this respect, it should be properly described and we should go with tools that help the customer financially estimate the amount of work required to achieve the desired quality level from a machine translation output.
Indeed, the PEMT effort depends on diverse factors such as the volume of content to process, the turnaround time, and the quality expectations for the finalized output. Most importantly, it depends on the suitability of source data and input for (machine) translation.
Therefore, however, assessable through automatic measurements, PEMT effort can only be loosely estimated and projected. In this respect, KantanMT is offering the finest tool combination for accurate estimates.
On the other hand, a downstream measurement of the PEMT effort by comparing the final post-edited translation with the raw machine translation output is reactive (just like the typical translation quality assessment practice) rather than predictive (that is business-oriented).
Also, a downstream compensation model requires an accurate measurement of the actual work performed to infer the percentage on the hourly rate from the edit distance, as no positive correlation exists between edit distance and actual throughput.
Nonetheless, tracking the PEMT effort can be useful if the resulting data is compared with estimates to derive a historical series. After all, that’s how data empowers us.
Predictability is a major driver in any business, and it should come as no surprise, then, that translation buyers have no interest in dealing with the intricacy of quality metrics that are irredeemably prone to subjectivity, ambiguity, and misinterpretation, and, most importantly, are irrelevant to them. When it comes to business — and real money — gambling is never the first option, but the last resort. (KV: Predictability here would mean a defined effort ($ & time) that would result in a defined outcome (sample of acceptable output)).
On the other hand, more than a quarter of a century has passed since the introduction of CAT tools in the professional field, many books and papers have been written about them, and yet many still feel the urge to explain what they are. Maybe this might make sense for the few customers who are entirely new to translation, even though what they could be interested to know is just that providers would use some tool of the trade and spare them some money. And yet, quality would remain a major concern, as a recent SDL study showed.
An introduction to CAT tools is at the very least curious when recipients are translation professionals or translation students about to graduate. Even debunking some still popular myths about CAT is just as curious, unless considering the number of preachers thundering from their virtual pulpits against the hazards of these instruments of the devil.
In this apocalyptic scenario, even a significant leap forward could go almost unnoticed. Lilt is an innovative translation tool, with some fabulous features, especially for professional translators. As Kirti Vashee points out, it is a virtual translator assistant. It also presents a few drawbacks, though.
Post-editing is the ferry to the singularity. It could be run interactively, or downstream on an entire corpus of machine translation output.
When fed with properly arranged linguistic data from existing translation memories, Lilt could be an extraordinary post-editing tool also on bilingual files. Unfortunately, the edits made by a single user only affects the dataset associated with that account and the task that is underway. In other words, Lilt is by no means a collaborative translation environment. Yet.
This means that, for Lilt to be effective with typically large PEMT jobs involving teams, accurate PEMT instructions are essential, and, most importantly, post-editors should strictly follow them. This is a serious issue. Computers never break rules, while free-will enables humans to deviate from them.
Finally, although cloud computing is now usual in business, Lilt can still present a major problem to many translation industry players for being only available in the cloud, due to the need for a fast Internet connection, or to the vexed — although repeatedly demystified — question of data protection for IP reasons, and despite the computing resources to process the vast amount of data that would hardly make sense for a typical SME to have.
In conclusion, when you start a business, it is usually to make money, and money is not necessarily bad if you do no evil, pecunia non olet. And money usually comes from buyers, whose prime requirement can be summarized as “Give me something I can understand.”
My ignorance will excuse me.
-----------------
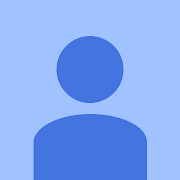
Luigi Muzii has been in the "translation business" since 1982 and has been a business consultant since 2002, in the translation and localization industry through his firm . He focuses on helping customers choose and implement best-suited technologies and redesign their business processes for the greatest effectiveness of translation and localization related work.
This link provides access to his other blog posts.