This is another, timely post describing the challenges of human quality assessment by Luigi Muzii. As we saw from the recent deceptive Google NMT announcements that while
there is a lot of focus on new machine learning approaches we are still using the same quality assessment approach of yesteryear: BLEU. Not much has
changed. It
is well understood that this metric is flawed but there seems to be no
useful replacement coming forward. This necessitates that some kind of human assessment also has to be made and invariably this human
review is also problematic. The best practices for these human assessments that I have seen are at Microsoft and eBay. The worst at many LSPs and Google. The key to
effective procedures seems to be, the presence of invested and objective linguists on the team, and a culture that has integrity and rigor without the
cumbersome and excessively detailed criteria that the "Translation Industry" seems to create (DQF & MDM for example). Luigi offers some insight on
this issue that I feel is worth note as we need to make as much more progress on the human assessment of MT output as well. Not only to restrain Google
from saying stupid shite like
“Nearly Indistinguishable From Human Translation” but also to really understand if we are making progress and understand better what needs to be
improved.
MT systems can only improve if competent linguistic feedback is provided as the algorithms will always need a "human" reference. The emphasis below is all mine and was not present in the original submission.
Dimensionally speaking, quality is a measurement, i.e. a figure obtained by measuring something.
Because of the intricacies related to the intrinsic nature of languages, objective measurement of translation quality has always been a much researched and debated topic that has borne very little fruit. The notion of understood quality level remains unsolved, together with any kind of generally accepted and clearly understood quality assessment and measurement.
Then along came machine translation and, since it’s inception, we have been facing the central issue of estimating the reliability and quality of MT engines. Quite obviously, this was done by comparing the quality of machine translated outputs to that of human reference data using statistical methods and models, or by having bilingual humans, usually, linguists, evaluate the quality of machine translated output.
Ad hoc algorithms based on specific metrics, like BLEU, were developed to perform automatic evaluation and produce an estimate of the efficiency of the engine for tuning and evaluation purposes. The bias implicit in the selection of the reference model remains a major issue, though, as there is not only one single correct translation. There can be many correct translations.
Human evaluation of machine translation has always been done in the same way as for human translations, with the same inconsistencies, especially when results are examined over time and when these evaluations are done by different people. The typical error-catching approach of human evaluation results is irredeemably biased, as long as errors are not defined uniquely and unambiguously, and if care is not taken to curb giving too much scope to the evaluator’s subjective preferences.
Indeed, human evaluation of machine translation is known for being expensive, time-consuming and often skewed, and yet it is supposed to overcome the drawbacks introduced by the limited accuracy and approximation of automatic evaluation. However, the complications of the many new quality measurement metrics proposed over the years have not yet reduced this rough approximation that we are still faced with. They have instead added to the confusion with these new metrics, which are not well understood and introduce new kinds of bias. In fact, despite the many efforts made over the last few years, the overall approach has remained the same, with a disturbing inclination to move in the direction of too much detail rather than move to more streamlined approaches. For example, the new complexity rising from the integration of DQF and MDM has proven to be expensive and unreliable so far and of limited value. Many know about the inefficiency and ineffectiveness of the SAE metrics once applied to the real world, with many new errors introduced by reviewers, together with many false positives. Indeed, translation quality metrics have become more and more complex and overly detailed, and always seem to be based on the error-catching approach that has proved costly and unreliable thus far. Automatic metrics can be biased too, especially when we assume that the human reference samples are human translation perfection, but at least they are fast, consistent, and convenient. And their shortcomings are widely known and understood.
People in this industry— and especially academics—seem to forget or ignore that every measurement must be of functional value to business, and that the plainer and simpler the measurement the better it is, enabling it to be easily grasped and easily used in a production mode.
On the other hand, just like human translation, machine translation is always of unknown quality, especially when rendered in a language unknown to the buyer, but it is intrinsically much more predictable and consistent, when compared to human translated projects with large batches of content, where many translators are possibly involved.
Effective upfront measurement helps to provide useful prior knowledge, thus reducing uncertainty, leading to well-informed decisions, and lessening the chance of deployment error. Ultimately, effective measurement helps to save money. Therefore, the availability of clear measures for rapid deployment are vital for any business using machine translation.
Also, any investment in machine translation is likely to be sizeable. Implementing a machine translation platform is a mid- to long-term effort requiring specialized resources and significant resilience to potentially frustrating outcomes in the interim. Effective measurements, including evaluation of outputs, provide a rational basis for selecting what improvements to make first.
In most cases, any measurement is only an estimate, a guess based on available information, made by approximation: it is almost correct and not intended to be exact.
Automatic scores give at least some idea of the efficiency and effectiveness of engines. This is crucial to estimate the distance from the required and expected level of performance, and the time for filling the gap.
For example, if using BLEU as the automatic assessment reference, 0.5-0.8 could be considered acceptable for full post-editing, 0.8 or higher for light post-editing.
Full post-editing consists in fixing machine-induced meaning (semantic) distortion, making grammatical and syntactic adjustments, checking terminology for untranslated terms that could possibly be new terms, partially or completely rewriting sentences for target language fluency. It is reserved for publishing and providing high quality input for engine training.
Light post-editing consists in adjusting mechanical errors, mainly for capitalization and punctuation, replacing unknown words, possibly misspelled in the source text, removing redundant words or inserting missing ones, and ignoring all stylistic issues. It is generally used for content to be re-used in different contexts, possibly through further adaptation.
Detailed analytics can also offer an estimate of where improvements, edits, adds, replacements, etc. must be made and this in turn helps in assessing and determining the effort required.
After a comprehensive analysis of automatic evaluation scores has been accomplished, machine translation outputs can then undergo human evaluation.
When coming to human evaluation, a major issue is sampling. In fact, to be affordable, human evaluation must be done on small portions of the output, which must be homogeneous and consistent with the automatic score.
Once consistent samples have been selected, human evaluation could start with fluency, which is affected by grammar, spelling, choice of words, and style. To prevent bias, evaluators must be given a predefined restricted set of criteria to comply with when voting/rating whether samples are fluent or not.
Fluency refers to the target only, without taking the source into account and its evaluation does not always require evaluators to be bilingual; indeed, it is often better that they are not. However, always consider that monolingual evaluation of target text only generally takes relatively short time, and judgments are generally consistent across different people, but that the more of instructions are provided to evaluators, the longer they take to complete their task, and the less consistent results are. Then the same samples would be passed to bilingual evaluators for adequacy evaluation.
Adequacy is defined as the amount of source meaning preserved in translation. This necessarily requires a comparative analysis of source and target texts, as adequacy can be affected by completeness, accuracy, and cleanup of training data. Consider using a narrow continuous measurement scale.
A typical pitfall of statistical machine translation is terminology. Human evaluation is useful to detect terminology issues. However, that could mean that hard work is required normalizing training data to realign terminology in each segment and analyze and amend translation tables.
Remember that, the number and magnitude of defects (errors) are not the best or the only way to assess quality in a translation service product. Perception can be equally important. When working with MT, in particular, the type and frequency of errors are pivotal, even though all these errors could not be all resolved. Take the Six Sigma model: what could be a reasonably expected level for an MT platform? Now take terminology in SMT, and possibly, in a near future, NMT. Will amending a very large training dataset be convenient to have the correct term(s) always used? Implementing and running an MT platform is basically a cost effectiveness problem. As we know, engines perform differently according to language pairs, amount, and quality of training data, etc.. This means that a one-size-fits-all approach for TQA is unsuitable, and waiving an engine from production use might be better than insisting in trying to use or improve it because the PEMT effort could be excessive. I don’t think that the existing models and metrics, including DQF, can be universally applied.
However, they could be helpful once automatic scores prove the engine could perform acceptably. In this case, defining specific categories for errors emerging from testing and operating engines that could potentially occur repeatedly is the right path to further engine tuning and development. And this can’t be made based on abstract and often abstruse (at least to non-linguists) metrics.
Finally, to get a useful PEMT effort indicator that provides an estimate of the work required for an editor to do to get the content over a predetermined acceptance quality level (AQL,) a weighted combination of correlation and dependence, precision and recall and edit distance scores can be computed. Anyway, the definition of AQLs is crucial for the effective implementation of a PEMT effort indicator, together with a full grasp of analytics, which requires an extensive understanding of the machine translation platform and the training data.
Many of these aspects, from a project management perspective, are covered in more detail in the TAUS PE4PM course.
This course also covers another important element of a post-editing project, the editor’s involvement and remuneration. Especially in the case of full post-editing, post-editors could be asked to contribute to train an engine, and editors could prove extremely valuable on the path to achieve better performances.
Last but not least, the suitability of source text for machine translation and the tools to use in post-editing can make the difference between success and failure in the implementation of a machine translation initiative.
When a post-editing job comes to an LSP or a translator, nothing can at that point be done on the source text or the initial requirements. Any action that can be taken must be taken upstream, earlier in the process. In this respect, while predictive quality analysis at a translated file level has already been implemented, although not fully substantiated yet, predictive quality analysis at source text level is still to come. It would be of great help to translation buyers in general who could base their investment on reasonable measures, possibly in a standard business logic, and possibly improve their content for machine translation and translatability in general. NLP research is already evolving to provide feedback on a user’s writing, reconstruct story lines or classify content, and assess style.
In terms of activities going on in the post-editing side of the world, adaptive machine translation will be a giant leap forward when every user’s edits are made available to an entire community, by permanently incorporating each user’s evolving datasets into the master translation tables. Thus the system is continuously improving with ongoing use in a way that other MT systems do not. At the moment, Adaptive MT is restricted to Lilt and SDL (all talk so far) users. This means that it won’t be available in corporate settings where MT is more likely to be implemented unless SDL software is already in use and/or IP is not an issue. Also, being very clear and objective about the rationale for implementing MT is essential to avoid being misled when interpreting and using analytics. Unfortunately, in most situations, this is not the case. For instance, if my main goal is speed, I should look into analytics for something other than what I should look for if my goal is cutting translation costs or increasing consistency. Anyway, understanding the analytics is no laughing matter. But this is another kettle of fish.
Luigi Muzii has been in the "translation business" since 1982 and has been a business consultant since 2002, in the translation and localization industry through his firm . He focuses on helping customers choose and implement best-suited technologies and redesign their business processes for the greatest effectiveness of translation and localization related work.
This link provides access to his other blog posts.
-------------
Dimensionally speaking, quality is a measurement, i.e. a figure obtained by measuring something.
Because of the intricacies related to the intrinsic nature of languages, objective measurement of translation quality has always been a much researched and debated topic that has borne very little fruit. The notion of understood quality level remains unsolved, together with any kind of generally accepted and clearly understood quality assessment and measurement.
Then along came machine translation and, since it’s inception, we have been facing the central issue of estimating the reliability and quality of MT engines. Quite obviously, this was done by comparing the quality of machine translated outputs to that of human reference data using statistical methods and models, or by having bilingual humans, usually, linguists, evaluate the quality of machine translated output.
Ad hoc algorithms based on specific metrics, like BLEU, were developed to perform automatic evaluation and produce an estimate of the efficiency of the engine for tuning and evaluation purposes. The bias implicit in the selection of the reference model remains a major issue, though, as there is not only one single correct translation. There can be many correct translations.
Human evaluation of machine translation has always been done in the same way as for human translations, with the same inconsistencies, especially when results are examined over time and when these evaluations are done by different people. The typical error-catching approach of human evaluation results is irredeemably biased, as long as errors are not defined uniquely and unambiguously, and if care is not taken to curb giving too much scope to the evaluator’s subjective preferences.
The problem
Indeed, human evaluation of machine translation is known for being expensive, time-consuming and often skewed, and yet it is supposed to overcome the drawbacks introduced by the limited accuracy and approximation of automatic evaluation. However, the complications of the many new quality measurement metrics proposed over the years have not yet reduced this rough approximation that we are still faced with. They have instead added to the confusion with these new metrics, which are not well understood and introduce new kinds of bias. In fact, despite the many efforts made over the last few years, the overall approach has remained the same, with a disturbing inclination to move in the direction of too much detail rather than move to more streamlined approaches. For example, the new complexity rising from the integration of DQF and MDM has proven to be expensive and unreliable so far and of limited value. Many know about the inefficiency and ineffectiveness of the SAE metrics once applied to the real world, with many new errors introduced by reviewers, together with many false positives. Indeed, translation quality metrics have become more and more complex and overly detailed, and always seem to be based on the error-catching approach that has proved costly and unreliable thus far. Automatic metrics can be biased too, especially when we assume that the human reference samples are human translation perfection, but at least they are fast, consistent, and convenient. And their shortcomings are widely known and understood.
People in this industry— and especially academics—seem to forget or ignore that every measurement must be of functional value to business, and that the plainer and simpler the measurement the better it is, enabling it to be easily grasped and easily used in a production mode.
On the other hand, just like human translation, machine translation is always of unknown quality, especially when rendered in a language unknown to the buyer, but it is intrinsically much more predictable and consistent, when compared to human translated projects with large batches of content, where many translators are possibly involved.
Effective upfront measurement helps to provide useful prior knowledge, thus reducing uncertainty, leading to well-informed decisions, and lessening the chance of deployment error. Ultimately, effective measurement helps to save money. Therefore, the availability of clear measures for rapid deployment are vital for any business using machine translation.
Also, any investment in machine translation is likely to be sizeable. Implementing a machine translation platform is a mid- to long-term effort requiring specialized resources and significant resilience to potentially frustrating outcomes in the interim. Effective measurements, including evaluation of outputs, provide a rational basis for selecting what improvements to make first.
In most cases, any measurement is only an estimate, a guess based on available information, made by approximation: it is almost correct and not intended to be exact.
In simple terms, the logic behind the evaluation of machine translation output is to get a few basic facts pinned down:
The efficiency and effectiveness of the MT engines;
The size of the effort required for further tuning the MT engine;
The extent and nature of the PEMT effort.
Each measure is related to one or more strategic decisions.
Automatic scores give at least some idea of the efficiency and effectiveness of engines. This is crucial to estimate the distance from the required and expected level of performance, and the time for filling the gap.
For example, if using BLEU as the automatic assessment reference, 0.5-0.8 could be considered acceptable for full post-editing, 0.8 or higher for light post-editing.
Full post-editing consists in fixing machine-induced meaning (semantic) distortion, making grammatical and syntactic adjustments, checking terminology for untranslated terms that could possibly be new terms, partially or completely rewriting sentences for target language fluency. It is reserved for publishing and providing high quality input for engine training.
Light post-editing consists in adjusting mechanical errors, mainly for capitalization and punctuation, replacing unknown words, possibly misspelled in the source text, removing redundant words or inserting missing ones, and ignoring all stylistic issues. It is generally used for content to be re-used in different contexts, possibly through further adaptation.
Detailed analytics can also offer an estimate of where improvements, edits, adds, replacements, etc. must be made and this in turn helps in assessing and determining the effort required.
After a comprehensive analysis of automatic evaluation scores has been accomplished, machine translation outputs can then undergo human evaluation.
When coming to human evaluation, a major issue is sampling. In fact, to be affordable, human evaluation must be done on small portions of the output, which must be homogeneous and consistent with the automatic score.
Once consistent samples have been selected, human evaluation could start with fluency, which is affected by grammar, spelling, choice of words, and style. To prevent bias, evaluators must be given a predefined restricted set of criteria to comply with when voting/rating whether samples are fluent or not.
Fluency refers to the target only, without taking the source into account and its evaluation does not always require evaluators to be bilingual; indeed, it is often better that they are not. However, always consider that monolingual evaluation of target text only generally takes relatively short time, and judgments are generally consistent across different people, but that the more of instructions are provided to evaluators, the longer they take to complete their task, and the less consistent results are. Then the same samples would be passed to bilingual evaluators for adequacy evaluation.
Adequacy is defined as the amount of source meaning preserved in translation. This necessarily requires a comparative analysis of source and target texts, as adequacy can be affected by completeness, accuracy, and cleanup of training data. Consider using a narrow continuous measurement scale.
A typical pitfall of statistical machine translation is terminology. Human evaluation is useful to detect terminology issues. However, that could mean that hard work is required normalizing training data to realign terminology in each segment and analyze and amend translation tables.
Remember that, the number and magnitude of defects (errors) are not the best or the only way to assess quality in a translation service product. Perception can be equally important. When working with MT, in particular, the type and frequency of errors are pivotal, even though all these errors could not be all resolved. Take the Six Sigma model: what could be a reasonably expected level for an MT platform? Now take terminology in SMT, and possibly, in a near future, NMT. Will amending a very large training dataset be convenient to have the correct term(s) always used? Implementing and running an MT platform is basically a cost effectiveness problem. As we know, engines perform differently according to language pairs, amount, and quality of training data, etc.. This means that a one-size-fits-all approach for TQA is unsuitable, and waiving an engine from production use might be better than insisting in trying to use or improve it because the PEMT effort could be excessive. I don’t think that the existing models and metrics, including DQF, can be universally applied.
However, they could be helpful once automatic scores prove the engine could perform acceptably. In this case, defining specific categories for errors emerging from testing and operating engines that could potentially occur repeatedly is the right path to further engine tuning and development. And this can’t be made based on abstract and often abstruse (at least to non-linguists) metrics.
Finally, to get a useful PEMT effort indicator that provides an estimate of the work required for an editor to do to get the content over a predetermined acceptance quality level (AQL,) a weighted combination of correlation and dependence, precision and recall and edit distance scores can be computed. Anyway, the definition of AQLs is crucial for the effective implementation of a PEMT effort indicator, together with a full grasp of analytics, which requires an extensive understanding of the machine translation platform and the training data.
Many of these aspects, from a project management perspective, are covered in more detail in the TAUS PE4PM course.
This course also covers another important element of a post-editing project, the editor’s involvement and remuneration. Especially in the case of full post-editing, post-editors could be asked to contribute to train an engine, and editors could prove extremely valuable on the path to achieve better performances.
Last but not least, the suitability of source text for machine translation and the tools to use in post-editing can make the difference between success and failure in the implementation of a machine translation initiative.
When a post-editing job comes to an LSP or a translator, nothing can at that point be done on the source text or the initial requirements. Any action that can be taken must be taken upstream, earlier in the process. In this respect, while predictive quality analysis at a translated file level has already been implemented, although not fully substantiated yet, predictive quality analysis at source text level is still to come. It would be of great help to translation buyers in general who could base their investment on reasonable measures, possibly in a standard business logic, and possibly improve their content for machine translation and translatability in general. NLP research is already evolving to provide feedback on a user’s writing, reconstruct story lines or classify content, and assess style.
In terms of activities going on in the post-editing side of the world, adaptive machine translation will be a giant leap forward when every user’s edits are made available to an entire community, by permanently incorporating each user’s evolving datasets into the master translation tables. Thus the system is continuously improving with ongoing use in a way that other MT systems do not. At the moment, Adaptive MT is restricted to Lilt and SDL (all talk so far) users. This means that it won’t be available in corporate settings where MT is more likely to be implemented unless SDL software is already in use and/or IP is not an issue. Also, being very clear and objective about the rationale for implementing MT is essential to avoid being misled when interpreting and using analytics. Unfortunately, in most situations, this is not the case. For instance, if my main goal is speed, I should look into analytics for something other than what I should look for if my goal is cutting translation costs or increasing consistency. Anyway, understanding the analytics is no laughing matter. But this is another kettle of fish.
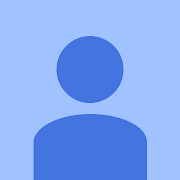
Luigi Muzii has been in the "translation business" since 1982 and has been a business consultant since 2002, in the translation and localization industry through his firm . He focuses on helping customers choose and implement best-suited technologies and redesign their business processes for the greatest effectiveness of translation and localization related work.
This link provides access to his other blog posts.
Very interesting.
ReplyDeleteBut is there really such need to go into the technical and statistical details of measuring translation quality?
By even dealing with such arguments are you not already making a concession to those who push the machine translation agenda?
I submit that only a qualified human translator can truly measure such quality.
Although determining criteria for evaluating the quality of translation by qualified human translators could be useful to eliminate or moderate the subjective aspect, determining criteria for machines is just a waste of time, unless of course you believe in the concept of what I guess you could call "machine quality", which I suppose could be useful in some specific contexts or perhaps for marketing purposes.
But as far as “quality” is concerned, with the absolute meaning a normal human being would normally give to that term, it can only be assessed by a qualified human translator.
Think about it if a machine were truly able to assess quality, then why couldn’t that machine be able to produce quality in the first place?
A very recent SDL study showed that the main cause for quality problems in translation was as follows:
ReplyDeleteThe study identifies the main cause of lack of quality as ‘terminology’ since this is the most frequent complaint. The proposed solutions are 1) terminology management, 2) the application of a formal standard such as ISO 9001 or LISA QA, and 3) an objective measurement of quality.
This is probably not different from a survey that could have taken place 5 or 10 years ago !!!
You can find more details here: http://www.sdl.com/cxc/language/translation-productivity/research-2016/